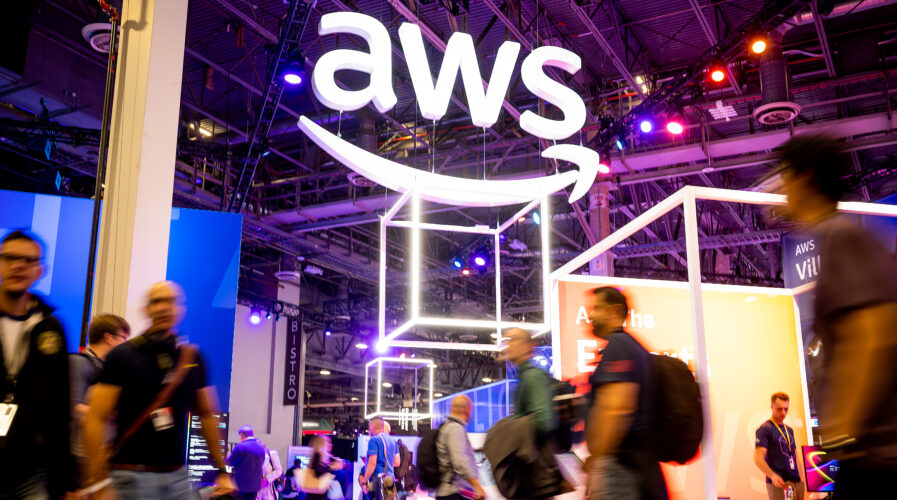
Announcements you might have missed from AWS reInvent 2022Source: AWS
Announcements you might have missed from AWS reInvent 2022
Cloud giant Amazon Web Service (AWS) has just concluded its 12th annual AWS re:Invent conference that took place last week in Las Vegas. As expected, as one of the signature events on the tech industry calendar, the reInvent that ran for four days was filled with many announcements covering a great deal of ground, especially in the world of cloud computing.
As is the case with most other large tech conferences, attendees at this year’s reInvent witnessed the cloud giant unveiling a multitude of new products and services, ranging from new data governance and sharing, to supply chain management services, as well as spatial simulation tools and capabilities, among others. AWS also showcased a series of new AI and machine learning offerings throughout reInvent.
Kickstarted with AWS CEO Adam Selipsky taking the stage on Tuesday to deliver his second reInvent keynote speech, he touched on the topics of water and energy production as well as consumption, but there was also an unmissable emphasis on data. Essentially, AWS is seizing an enormous opportunity as organizations continue to produce more data than ever, by providing integrations and other capabilities to make extraction and analysis easier.
Throughout his two-hour reInvent address, he had launched 10 new products—from the new AWS Supply Chain and Amazon DataZone to AWS SimSpace Weaver and new EC2 Hpc6id Instances. More details on Selipsky’s keynote can be found here. There were of course more to just what Selipsky unveiled. Let’s dive into that.
Democratizing AI and ML with SageMaker
Basically, AWS showcased its expanded lineup of artificial intelligence capabilities through its enhanced version of SageMaker, its neural network development platform. Originally introduced in 2017, SageMaker includes more than a dozen AI development tools. To put it simply, software teams, “tens of thousands of them”, as unveiled by AWS, use the platform to create neural networks, train them, monitor their performance after they’re deployed and perform related tasks.
“Many customers are using ML at a scale that was unheard of just a few years ago,” AWS VP of AI and ML Bratin Saha said. “The new Amazon SageMaker capabilities announced today make it even easier for teams to expedite the end-to-end development and deployment of ML models.” When it comes to AI governance, the first tool unveiled is the Amazon SageMaker Role Manager, which allows administrators to regulate user access more easily to a company’s SageMaker environment. Administrators can configure who can access what SageMaker features and how through a centralized console.
Then there is a newly added AI governance tool, Amazon SageMaker Model Cards, which will help software teams manage the data produced as part of machine learning projects. That data includes items such as AI training datasets and the results of neural network reliability tests. According to AWS, SageMaker Model Cards enable engineers to store such information in a centralized location for easy access.
Rounding out the lineup of AI governance features for SageMaker is the Amazon SageMaker Model Dashboard. It provides a console for monitoring the reliability of AI models after they’re deployed in production. The tool can help administrators detect and more quickly fix errors such as a sudden decline in the accuracy of an AI application’s processing results.
Refining data in AI projects
SageMaker also includes a tool called Amazon SageMaker Studio Notebooks that developers can use to create new neural networks. During the reInvent, AWS announced that SageMaker Studio Notebooks is receiving a new feature that can help developers spot errors in the data they process as part of AI projects.
According to AWS, the feature identifies data quality issues and recommends ways to remediate them. When a developer selects one of the suggested remediation methods, the tool automatically generates the software code necessary to implement it. And since, in an organization, AI applications are often developed by multiple software teams, SageMaker Studio Notebooks also added a new collaboration feature that will make it easier for teams to share AI model code and other software components with one another.
Geospatial ML in SageMaker
Certainly, the cornerstone of the AWS AI/ML portfolio is the SageMaker suite of services. “Tens of thousands of customers are using SageMaker ML models to make more than a trillion predictions a month,” AWS’ VP for database, analytics and ML, Swami Sivasubramanian claimed during his keynote address at the reInvent.
“Our customers are solving complex problems with SageMaker by using that data to build ML models ranging from optimizing driving routes for rideshare apps to accelerating drug discovery.” He then shared how geospatial data can be used for a wide variety of use cases, such as to optimize an agricultural harvest yield, assisting with planning for sustainable urban development or even to identify a new location or region for a business to open.
“Accessing high-quality geospatial data to train ML models requires working with multiple data sources and multiple vendors,” he said. “These data sets are typically massive and unstructured, which needs time consuming data preparation before you can even start writing a single line of code to build your ML models.” With the new geospatial support in SageMaker, Sivasubramanian said AWS aims to make it easier for organizations to actually build and deploy models.
READ MORE
- 3 Steps to Successfully Automate Copilot for Microsoft 365 Implementation
- Trustworthy AI – the Promise of Enterprise-Friendly Generative Machine Learning with Dell and NVIDIA
- Strategies for Democratizing GenAI
- The criticality of endpoint management in cybersecurity and operations
- Ethical AI: The renewed importance of safeguarding data and customer privacy in Generative AI applications